Non-Convex Total Variation Regularization for ill-posed Inverse Problems
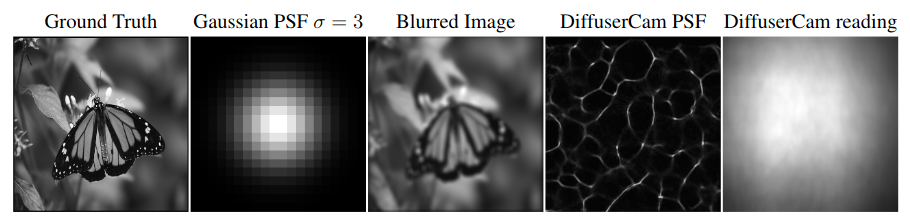
Contributors
Anirudh Aatresh, Praveen Pokala, Dr. Chandra Sekhar Seelamantula.
Summary
Lensless cameras are a new class of vision systems that can capture an image of a scene without the presence of a lens. In contrast to conventional lens-based vision systems, images of the scene in a lensless camera are found by solving an inverse problem, amounting to deconvolution and image reconstruction. In this project, we studied two such cameras, Diffusercam and Flatcam. After a single-step calibration process, these cameras capture a scene's image by computing the solution to an ill-posed inverse problem. The quality of the solution is influenced by the type of regularization used.
Total-variation (TV) regularization is often preferred to obtain a stable solution for ill-posed inverse problems in computational imaging. TV regularization promotes sparsity in the gradient domain and favors piece-wise constant reconstructions. However, convex TV schemes introduce bias in the amplitude estimates because they attach equal importance to all the gradient components. In this project, we proposed using non-convex TV minimization instead of the standard isotropic/anisotropic convex TV minimization. Non-convex TV minimization overcomes the bias introduced in standard TV minimization approaches. As the proposed non-convex TV penalty does not have a closed-form proximal operator, we developed an iteratively reweighted anisotropic TV minimization strategy for solving the problem under consideration. We demonstrate the efficacy of the proposed framework considering the application to image deblurring and the reconstruction problem in lensless imaging systems such as DiffuserCam and FlatCam.