LiverNet
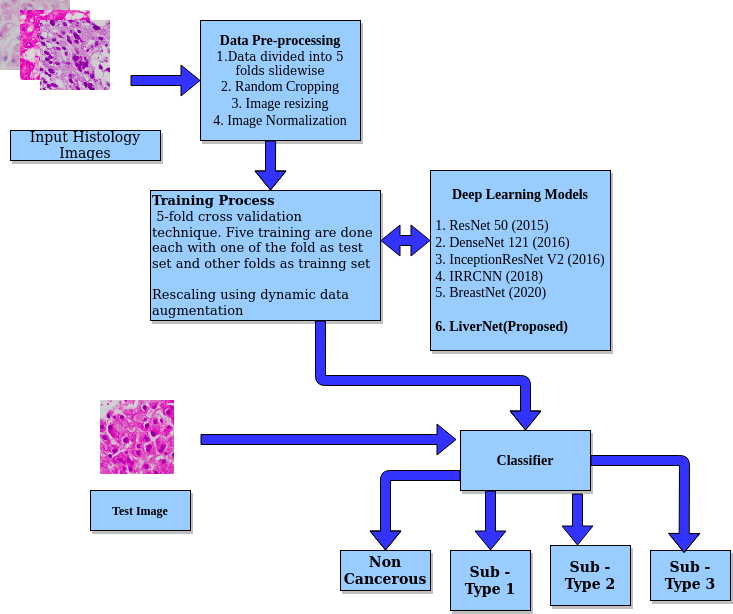
Contributors
Anirudh Aatresh, Kumar Alabhya, Dr. Shyam Lal, Dr. Jyoti Kini.
Summary
Manual liver hepatocellular carcinoma (HCC) grading is laborious and error-prone. In this project, we proposed a deep learning framework to automate HCC tumors' classification using their H&E stained histopathology images. Inspired by the success of convolution block attention modules (CBAM) and atrous spatial pyramid pooling (ASPP), we developed a novel neural network architecture that achieves approximately 2% improvement in classification accuracy and F1 score while requiring only a fraction of the computation and memory, compared to other state-of-the-art methods at the time. We tested our method on two datasets, a newly created dataset of our own - the KMC dataset and the TCGA dataset.
This work was published in the International Journal of Computer Assisted Radiology and Surgery in 2021.